Dive into the brief overviews of our final two poster-worthy research abstracts at SLEEP 2021
From new and even larger clinical validation results on our AI scoring, to enhancements to our AI’s capabilities, our research team dove deep into the data to discover how we can continue to find ways to make a positive impact on the world of sleep medicine. If you missed our first four abstracts in part one, make sure to jump over and give that a read, as well! But with that said, let’s dive into our final two abstracts.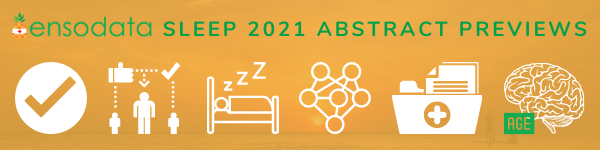
Clinical Validation of AI Scoring in Adult and Pediatric Patients
We would be crazy not to continue improving our AI sleep scoring solution, and while we don’t want to bore you with numbers, this is that point in the adventure. In our “Clinical Validation of AI Scoring in Adult and Pediatric Clinical PSG Samples Compared to Prospective, Double-Blind Scoring Panel” study, our team sought to set a new standard for AI-assisted sleep scoring and staging. For many doubters, there is still not enough data validation on the ability of AI in sleep scoring. To that end, we conducted the largest ever study of AI’s ability to analyze sleep, which includes roughly 3 times more data points than the next largest sleep study of this kind, (that we can find – if we’re wrong, please let us know.) The dataset is also very diverse, with a wide variety of demographic differences, including age, gender, BMI, medical conditions, medications, and other characteristics. Using this massive data set, we put EnsoSleep to work. And the numbers speak for themselves. You can see in the table below, that the agreement levels are quite impressive.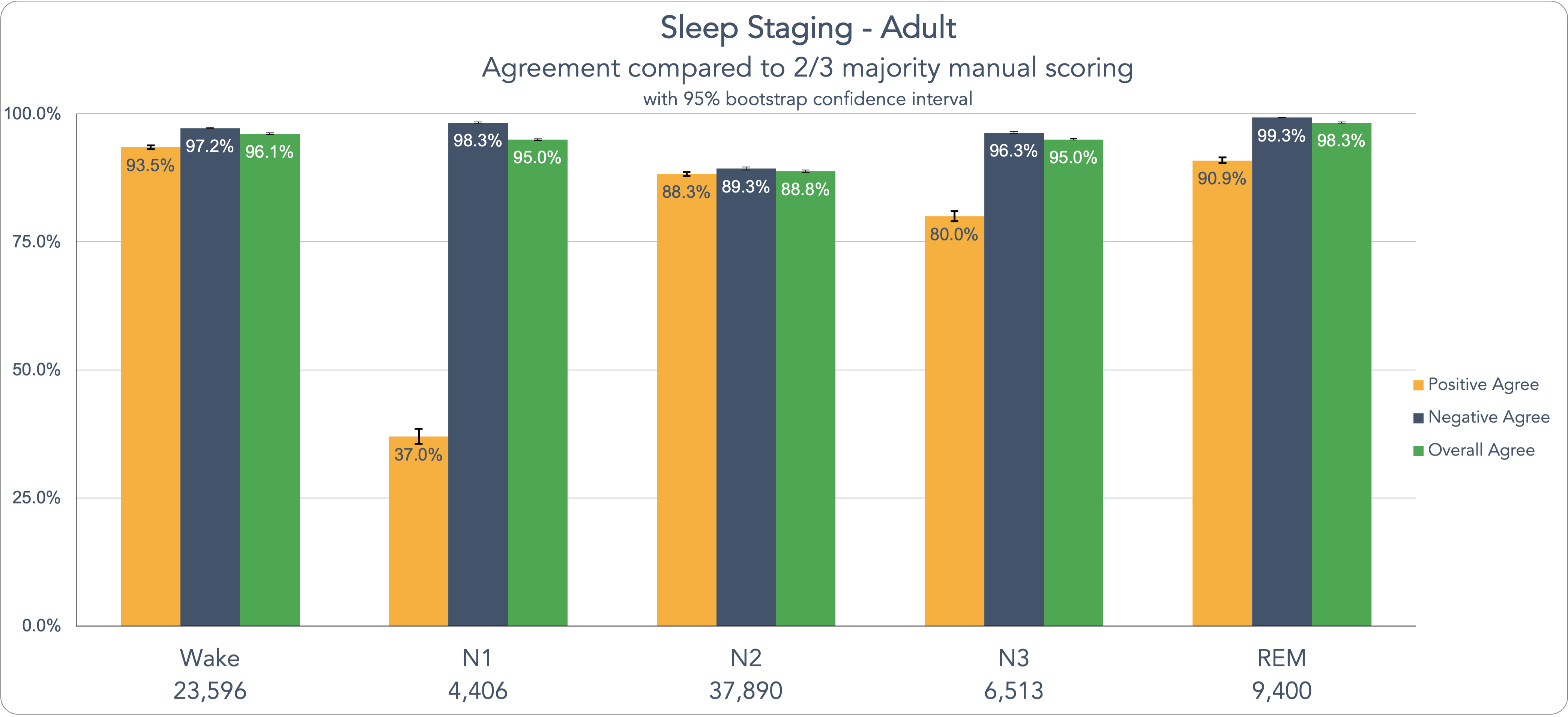
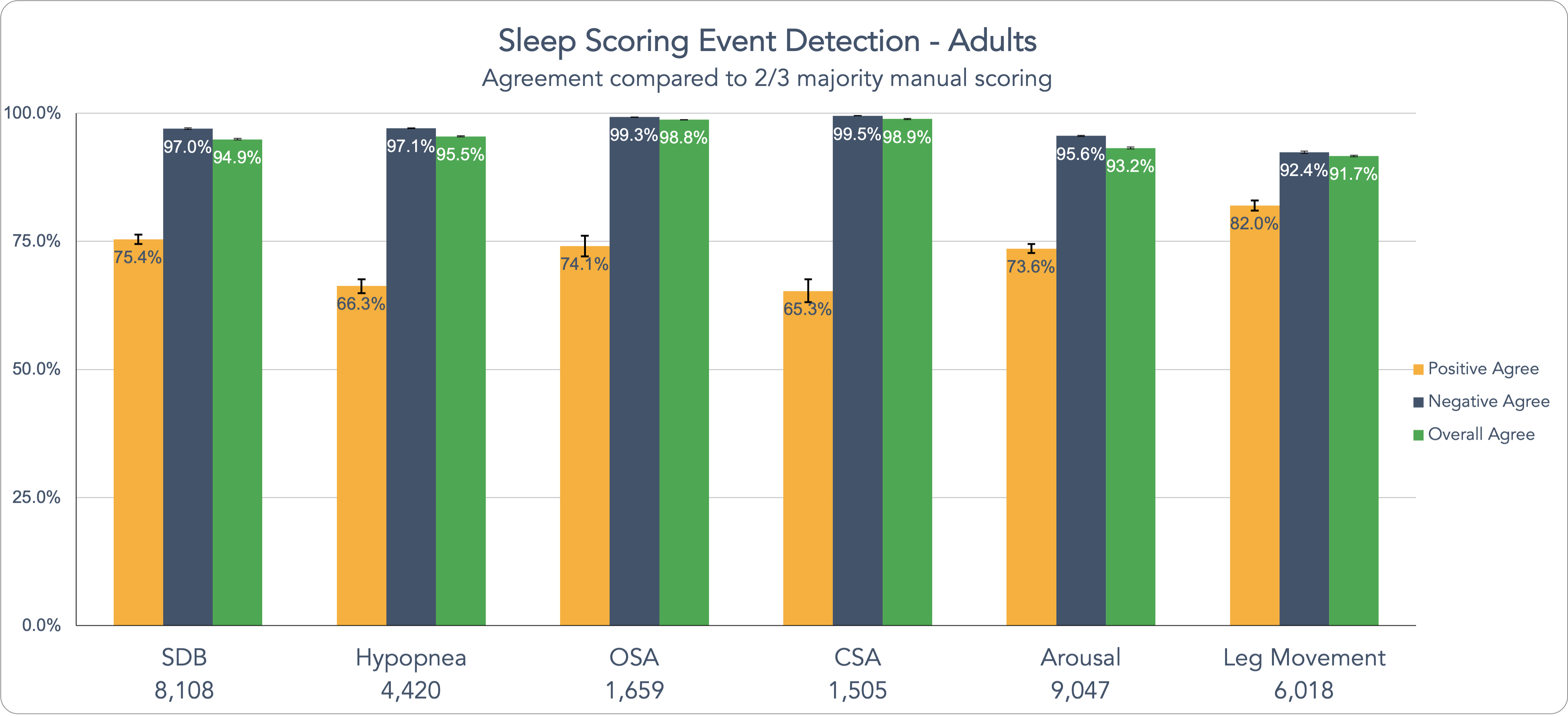