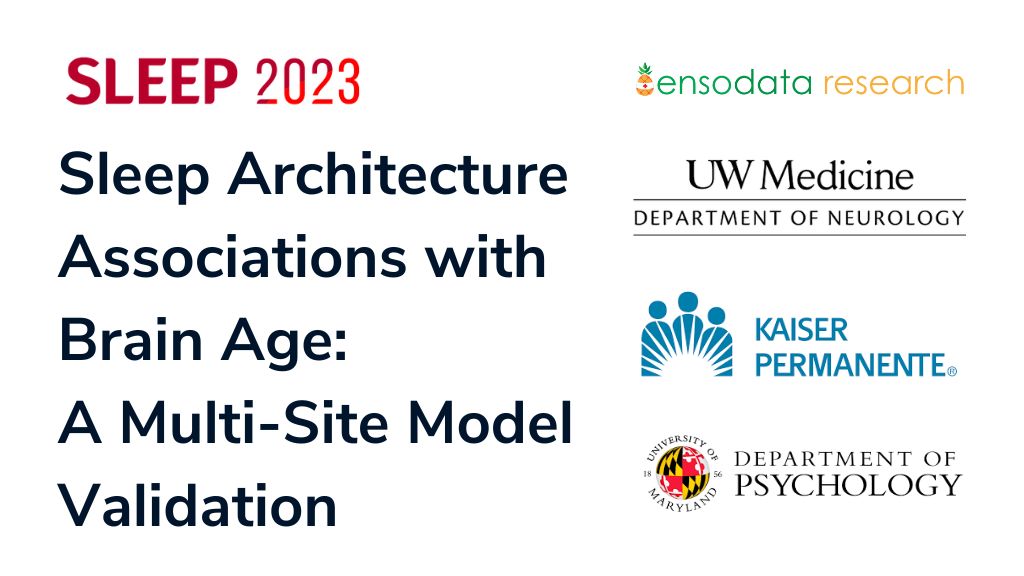
Sleep Architecture Associations with Brain Age: A Multi-Site Model Validation
This research study evaluates large multi-site datasets and assesses the relationship of N3/REM sleep duration with the predicted brain age.
This research study evaluates large multi-site datasets and assesses the relationship of N3/REM sleep duration with the predicted brain age.
In this study, EnsoData shows how ML algorithms based on PAP usage can predict future adherence, offering potential for personalized treatment decisions and preemptive interventions when upcoming non-adherence is predicted.
This research study demonstrated that Machine Learning methods can automatically detect Type I Narcolepsy using in PSG-EEG with promising degrees of accuracy.
This study demonstrates the ability of AI approaches produced high specificity and moderate sensitivity for REM Behavior Disorder and the potential to expand early detection and diagnosis of RBD.
This research study shows how AI can deliver strong predictive performance for PAP adherence within the first few weeks of therapy, enabling early PAP intervention or transition to alternative therapies sooner in the process and improving patient outcomes.
In this study, we evaluate whether patients are likely to comply with receiving a follow-up PSG following an indeterminate HSAT to rule out any presence of OSA and assess the demographic characteristics of individuals who are more likely to follow the AASM guidelines.
This research examined the feasibility for machine learning algorithms to improve upon screening for obstructive and central sleep apnea (SA) at the population health level using existing health insurance claims data.
In this study, we contribute to this growing body of clinical AI validation evidence and experimental design methodologies with an interoperable AI scoring engine for sleep studies in Adult and Pediatric populations.
In this study, we clinically validate the performance of AI for interoperable, PPG-based sleep staging and sleep disordered breathing event detection.
This study looks to identify CPAP adherence phenotypes in order to assist with the creation of more precise and personalized patient categorization and treatment.